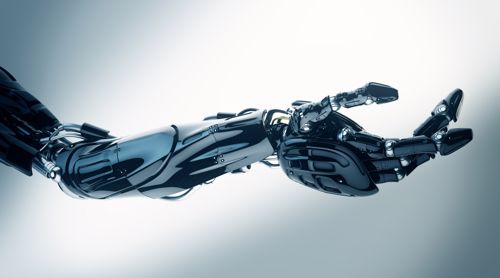
Reinforcement learning and AI implementation
By Max BurkhalterAugust 8, 2022
Reinforcement learning is defined as the subsect of machine learning (ML) where data scientists focus on decision-making and reward-based training. Put simply, there is a science to rationalization and determination. This article will examine if today's artificial intelligence (AI) and ML systems can wield this ability to impact the evolution of computer systems.
Like us
Reinforcement learning allows machines to mimic the way humans learn. Positive reinforcement isn't always the end result of our collective inquisitiveness, and the mistakes we make along the way shape the way we evolve our critical thinking skills. Essentially, reinforcement learning constructs a 'trial and error' methodology into AI and ML protocols – resulting in a continually layered determination algorithm for our advanced computational models.
Synopsys details the process behind allowing interpretation to influence machine learning. Consider their example: In a learning environment where a supervisor is removed, the learner must independently discover the correct path of actions that maximize the desired reward.
This discovery process is essentially an algorithm-based trial-and-error search, where the quality of these determinations is measured not only by the presence of an immediate reward but by the possibility of a delayed reward as well.
As ML systems can learn the actions that result in eventual success within an unseen and unstructured environment – without the help of a supervisor – reinforcement learning becomes a very powerful behavior.
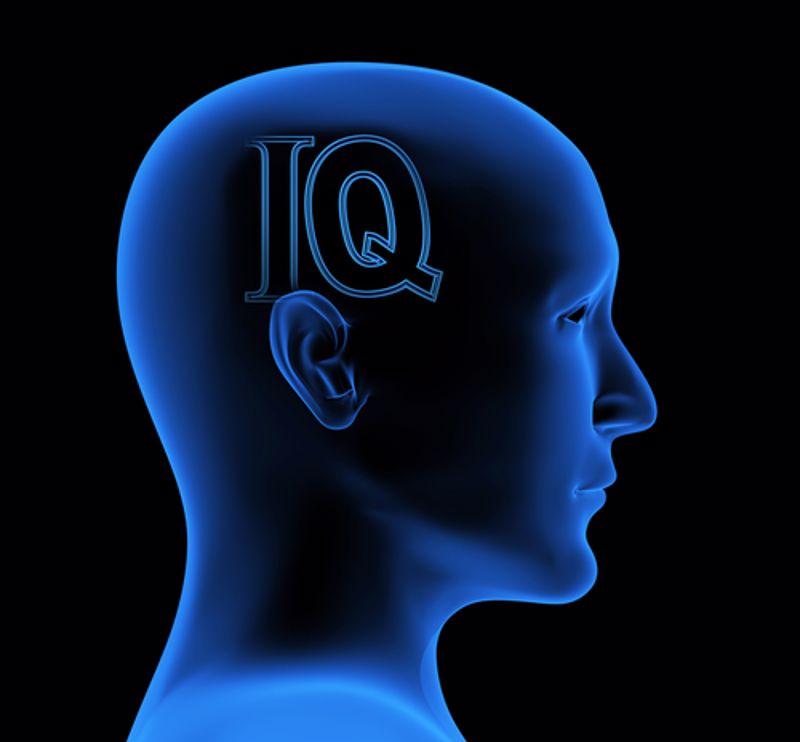
Reinforcing AI decision-making
A Daisy Intelligence report discusses the application of this exciting technology for today's already-robust AI learning environments. Theoretically, an AI system could run 100 million hours of simulation time in an hour of real-time with the presence of an advanced edge computing platform.
This process essentially empowers a series of decisions that have never been attempted by humans, due to the fact that real-time decision-making algorithms can be executed without historical decisions to interpret from.
The freedom from the math of predictive modeling presents exciting opportunities for AI architectures.
As explained in the aforementioned report, a predictive model is only able to replicate what has been previously accomplished and cannot learn new patterns without referring to examples. This limitation make AI and ML-powered learning behaviors inferior to human processing due to the restrictive modeling of mimicking human patterns.
Reinforcement learning, however, frees AI and ML systems to find completely new alternatives we collectively could never comprehend, as they exist beyond our rational thought limitations.
The future modeling capabilities of computer intelligence are virtually limitless thanks to exciting ML developments like reinforcement learning.
Reliable solutions
Perle stands ready to provide efficient, reliable technologies in order to adapt to the needs of constantly-innovating AI and ML powered industries. Learn more about how Perle is providing industrial servers for companies harnessing next-generation artificial intelligence solutions by contacting us today.